MPU6050数据滤波方式
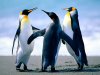
2楼
/** * IIR filter the samples. */ int16_t iirLPFilterSingle(int32_t in, int32_t attenuation, int32_t* filt) { int32_t inScaled; int32_t filttmp = *filt; int16_t out; if (attenuation > (1<<IIR_SHIFT)) { attenuation = (1<<IIR_SHIFT); } else if (attenuation < 1) { attenuation = 1; } // Shift to keep accuracy inScaled = in << IIR_SHIFT; // Calculate IIR filter filttmp = filttmp + (((inScaled-filttmp) >> IIR_SHIFT) * attenuation); // Scale and round out = (filttmp >> 8) + ((filttmp & (1 << (IIR_SHIFT - 1))) >> (IIR_SHIFT - 1)); *filt = filttmp; return out; }
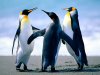
4楼
贴一下以前做的卡尔曼滤波的程序,比较简单的arduino程序:
void loop() { /* Update all the values */ while(i2cRead(0x3B,i2cData,14)); accX = ((i2cData[0] << 8) | i2cData[1]); accY = ((i2cData[2] << 8) | i2cData[3]); accZ = ((i2cData[4] << 8) | i2cData[5]); tempRaw = ((i2cData[6] << 8) | i2cData[7]); gyroX = ((i2cData[8] << 8) | i2cData[9])+112; gyroY = ((i2cData[10] << 8) | i2cData[11])+69; gyroZ = ((i2cData[12] << 8) | i2cData[13])+112; // atan2 outputs the value of -π to π (radians) - see http://en.wikipedia.org/wiki/Atan2 // We then convert it to 0 to 2π and then from radians to degrees accXangle = (atan2(accY,accZ)+PI)*RAD_TO_DEG; accYangle = (atan2(accX,accZ)+PI)*RAD_TO_DEG; double gyroXrate = (double)gyroX/131.0; double gyroYrate = -((double)gyroY/131.0); gyroXangle += gyroXrate*((double)(micros()-timer)/1000000); // Calculate gyro angle without any filter gyroYangle += gyroYrate*((double)(micros()-timer)/1000000); //gyroXangle += kalmanX.getRate()*((double)(micros()-timer)/1000000); // Calculate gyro angle using the unbiased rate //gyroYangle += kalmanY.getRate()*((double)(micros()-timer)/1000000); compAngleX = (0.93*(compAngleX+(gyroXrate*(double)(micros()-timer)/1000000)))+(0.07*accXangle); // Calculate the angle using a Complimentary filter compAngleY = (0.93*(compAngleY+(gyroYrate*(double)(micros()-timer)/1000000)))+(0.07*accYangle); kalAngleX = kalmanX.getAngle(accXangle, gyroXrate, (double)(micros()-timer)/1000000); // Calculate the angle using a Kalman filter kalAngleY = kalmanY.getAngle(accYangle, gyroYrate, (double)(micros()-timer)/1000000); timer = micros(); temp = ((double)tempRaw + 12412.0) / 340.0; /* Print Data */ /* Serial.print(accX);Serial.print(","); /* Serial.print(accY);Serial.print(","); Serial.print(accZ);Serial.print(","); Serial.print(gyroX);Serial.print(","); Serial.print(gyroY); Serial.print(","); Serial.print(gyroZ);Serial.print(","); */ Serial.print(accXangle);Serial.print(","); Serial.print(gyroXangle);Serial.print(","); Serial.print(compAngleX);Serial.print(","); Serial.print(kalAngleX);Serial.print(", "); Serial.print(""); Serial.print(accYangle);Serial.print(","); Serial.print(gyroYangle);Serial.print(","); Serial.print(compAngleY); Serial.print(","); Serial.print(kalAngleY);Serial.print(","); //Serial.print(temp);Serial.print(","); Serial.println(""); delay(10); }
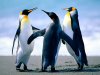
5楼
上面的程序中的卡尔曼滤波函数也贴上来,需要的拿去:
/* Copyright (C) 2012 Kristian Lauszus, TKJ Electronics. All rights reserved. This software may be distributed and modified under the terms of the GNU General Public License version 2 (GPL2) as published by the Free Software Foundation and appearing in the file GPL2.TXT included in the packaging of this file. Please note that GPL2 Section 2[b] requires that all works based on this software must also be made publicly available under the terms of the GPL2 ("Copyleft"). Contact information ------------------- Kristian Lauszus, TKJ Electronics Web : http://www.tkjelectronics.com e-mail : kristianl@tkjelectronics.com */ #ifndef _Kalman_h #define _Kalman_h class Kalman { public: Kalman() { /* We will set the varibles like so, these can also be tuned by the user */ Q_angle = 0.001; Q_bias = 0.003; R_measure = 0.03; bias = 0; // Reset bias P[0][0] = 0; // Since we assume tha the bias is 0 and we know the starting angle (use setAngle), the error covariance matrix is set like so - see: http://en.wikipedia.org/wiki/Kalman_filter#Example_application.2C_technical P[0][1] = 0; P[1][0] = 0; P[1][1] = 0; }; // The angle should be in degrees and the rate should be in degrees per second and the delta time in seconds double getAngle(double newAngle, double newRate, double dt) { // KasBot V2 - Kalman filter module - http://www.x-firm.com/?page_id=145 // Modified by Kristian Lauszus // See my blog post for more information: http://blog.tkjelectronics.dk/2012/09/a-practical-approach-to-kalman-filter-and-how-to-implement-it // Discrete Kalman filter time update equations - Time Update ("Predict") // Update xhat - Project the state ahead /* Step 1 */ rate = newRate - bias; angle += dt * rate; // Update estimation error covariance - Project the error covariance ahead /* Step 2 */ P[0][0] += dt * (dt*P[1][1] - P[0][1] - P[1][0] + Q_angle); P[0][1] -= dt * P[1][1]; P[1][0] -= dt * P[1][1]; P[1][1] += Q_bias * dt; // Discrete Kalman filter measurement update equations - Measurement Update ("Correct") // Calculate Kalman gain - Compute the Kalman gain /* Step 4 */ S = P[0][0] + R_measure; /* Step 5 */ K[0] = P[0][0] / S; K[1] = P[1][0] / S; // Calculate angle and bias - Update estimate with measurement zk (newAngle) /* Step 3 */ y = newAngle - angle; /* Step 6 */ angle += K[0] * y; bias += K[1] * y; // Calculate estimation error covariance - Update the error covariance /* Step 7 */ P[0][0] -= K[0] * P[0][0]; P[0][1] -= K[0] * P[0][1]; P[1][0] -= K[1] * P[0][0]; P[1][1] -= K[1] * P[0][1]; return angle; }; void setAngle(double newAngle) { angle = newAngle; }; // Used to set angle, this should be set as the starting angle double getRate() { return rate; }; // Return the unbiased rate /* These are used to tune the Kalman filter */ void setQangle(double newQ_angle) { Q_angle = newQ_angle; }; void setQbias(double newQ_bias) { Q_bias = newQ_bias; }; void setRmeasure(double newR_measure) { R_measure = newR_measure; }; private: /* Kalman filter variables */ double Q_angle; // Process noise variance for the accelerometer double Q_bias; // Process noise variance for the gyro bias double R_measure; // Measurement noise variance - this is actually the variance of the measurement noise double angle; // The angle calculated by the Kalman filter - part of the 2x1 state matrix double bias; // The gyro bias calculated by the Kalman filter - part of the 2x1 state matrix double rate; // Unbiased rate calculated from the rate and the calculated bias - you have to call getAngle to update the rate double P[2][2]; // Error covariance matrix - This is a 2x2 matrix double K[2]; // Kalman gain - This is a 2x1 matrix double y; // Angle difference - 1x1 matrix double S; // Estimate error - 1x1 matrix }; #endif
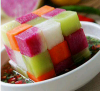
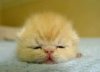
[無責任發言]
基本上, IIR 濾波是(頻域)濾波器, Kalman則是(時域)濾波器. 沒有誰好誰壞之分, 端看應用面.
IIR 較相近是對某固定主頻率的(低通)濾波器. 這個適合在SENSOR上有雜訊時, 把NOISE濾掉. 在MUP6050上而言, 雜訊就是當你讓SENSOR 本身靜止不動時, 但若它卻送出抖動的訊息時, 那就是雜訊.
Kalman則是非固定的, 它可以隨時間收集到的DATA, 做出預測及動態調整要做濾波的主頻率, 所以特別適合用在追蹤某目標的位置/速度/加速度時, 對目標的位置估計(去掉NOISE)
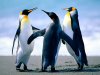
9楼
看过几个视频,关于卡尔曼滤波,互补滤波的对比视频,发现卡尔曼滤波速度还是快一点?或者是互补滤波过滤的有点过了?
视频地址http://v.youku.com/v_show/id_XNTQyODUxNzU2.html
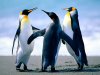
10楼
嗯,学习了,非专业菜鸟,看到两个滤波以为是一种东西,贻笑大方了。在上面的卡尔曼滤波中,并没有对传感数据进行滤波,而是直接求角度后卡尔曼滤波,结果也是很准确,比较好的反映了角度。在我们的四轴上是不是也可以这样处理呢?不用对采集的acc和角速度处理,而是求角度后再卡尔曼滤波?
回复
打赏帖 | |
---|---|
【S32K146】S32DS watchdog 配置使用被打赏20分 | |
【Zephyr】使用 IAR 调试 Zephyr 镜像被打赏20分 | |
【Zephyr】MCXN947 Zephyr 开发入门适配shell被打赏20分 | |
【我要开发板】6.联合MATLAB记录数据被打赏50分 | |
【瑞萨RA2E1开发板】:使用ADC功能实现位移传感器采集方案被打赏20分 | |
【nRF7002DK】基于sht30的温湿度计被打赏20分 | |
【nRF7002DK】日志打印被打赏20分 | |
【换取手持示波器】RGB屏幕移植ARM-2D库被打赏35分 | |
【分享开发笔记,赚取电动螺丝刀】分享一下如何解决瑞萨RA2E1使用printf编译报错问题被打赏27分 | |
rtthread硬件加密-5hash加密分析被打赏10分 |